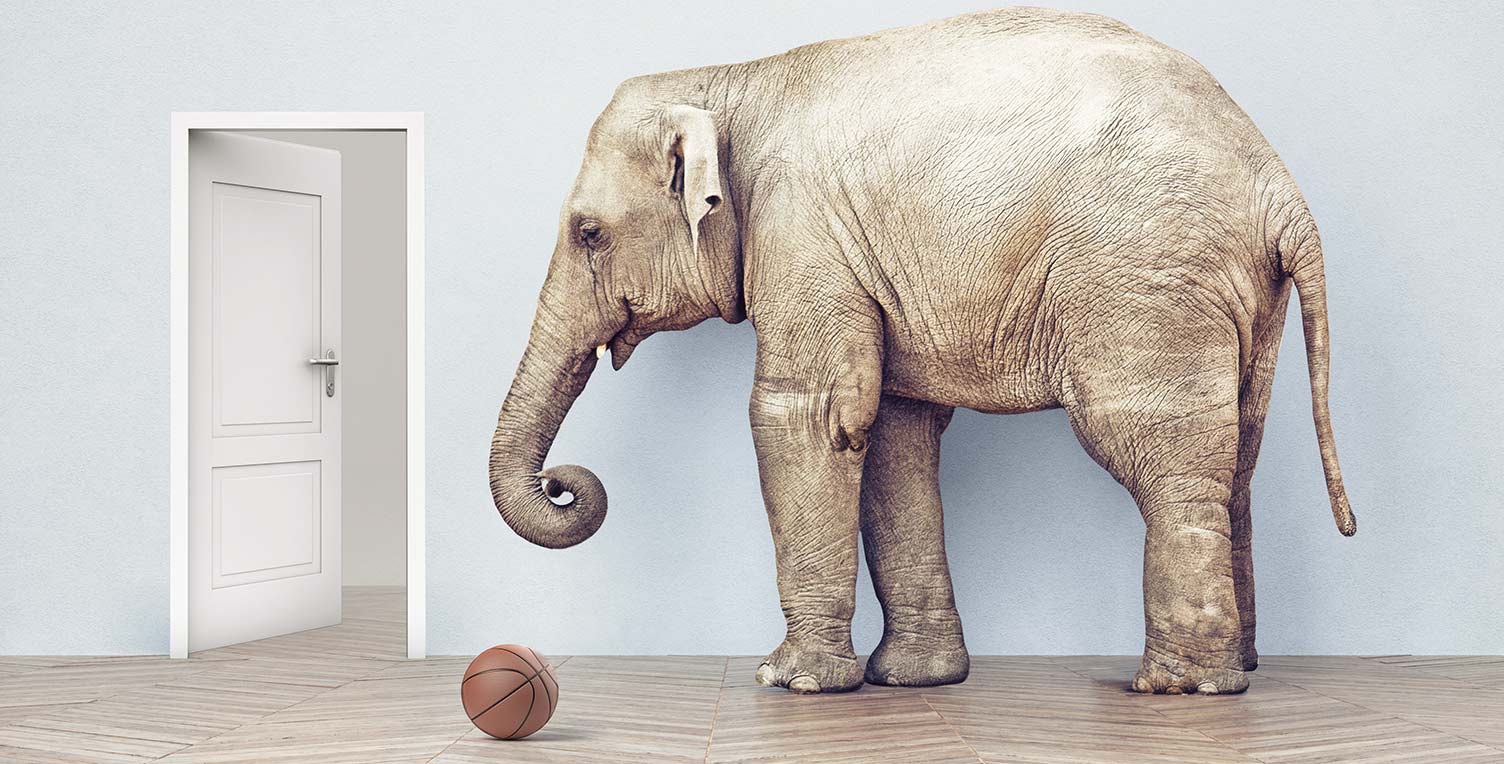
The Multi-modal Open World Grounded Learning and Inference (MOWGLI) project is our commonsense reasoning project. The goal is to build a system that can answer a wide range of common sense questions posed using either an image or natural language, about everyday intuitive phenomena such as abduction, analogy, causality, agency, physics, and social interactions. Our approach combines knowledge graphs (KG) and language models in novel ways to leverage the detailed knowledge contained in knowledge graphs and the robustness of language models. To that end we are building a first of its kind Commonsense Knowledge Graph (CSKG) that integrates existing commonsense KGs such as ConceptNet and Atomic, lexical resources such as Wordnet, Roget and FrameNet/VerbNet, factual KGs such as Wikidata, and other resources such as VisualGenome. We are investigating reasoning algorithms that use language models and deep learning to reason over the CSKG to produce explainable answers to commonsense questions. We are collaborating with Jure Leskovec at Stanford, Sameer Singh at UCI, Deborah McGuinness at RPI and Henry Lieberman at MIT.
MOWGLI is a project in the DARPA MCS program, supported by United States Office Of Naval Research under Contract No. N660011924033